ICDPR 2025 Speaker
Sidebar
Organized by
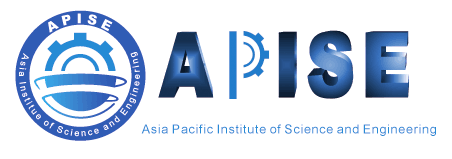
Sponsored by
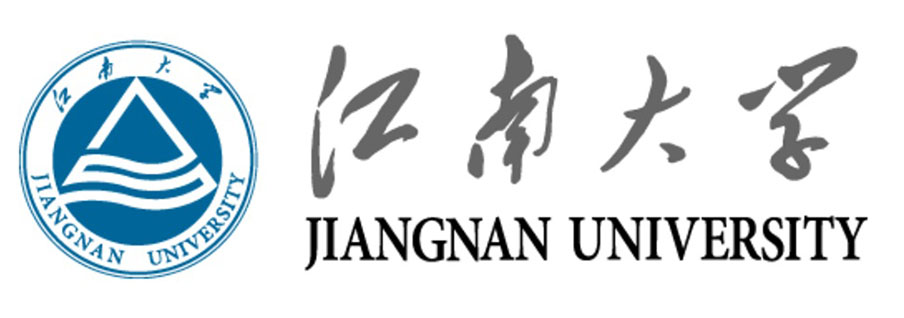
Technically Sponsored by
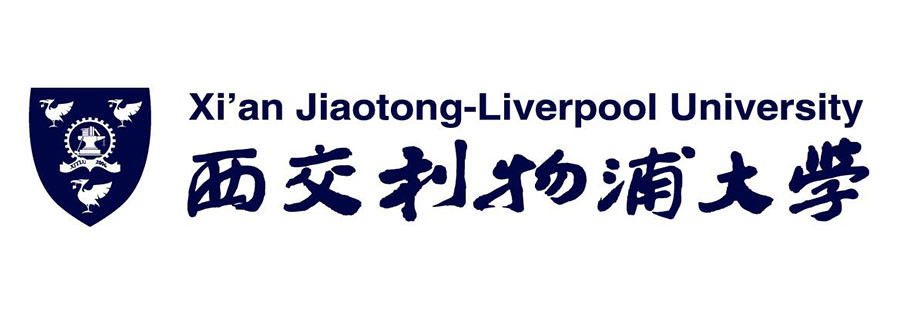
APISE Official Wechat
APISE Official Linkedin
APISE WeChat (for Consultation)
Conference Secretary:
Ms. Cici Chou
Phone: +86-19102815802 (Mainland China)
Wechat: APISE17358663189
Email: icdpr@hysdces.com
Prof. Sos Agaian
The City University of New York, USA
Fellow IEEE
(Keynote Speaker)
Speech Title: Bio-Inspired Single-Image Quality Assessment: Bridging Human and Computer Vision
Abstract: Bio-inspired image processing draws from computational neuroscience, cognitive science, and biology to develop algorithms for real-world image processing systems, enabling computers to "see" as humans do. This interdisciplinary approach has led to the creating of various image-processing algorithms with different levels of alignment to biological vision studies. However, digital images undergo various distortions during acquisition, processing, transmission, compression, storage, and reproduction. How can we accurately and efficiently measure the quality of a single image, even without a reference?
This talk explores the cutting-edge perception-guided single-image quality assessment (IQA) field. We delve into how bio-inspired computation, drawing inspiration from human visual perception, revolutionizes IQA. We will cover (i) how models based on biological vision systems can provide novel, robust, and computationally efficient ways to assess image quality, (ii) a synopsis of the latest research and breakthroughs in single-image "blind" IQA, (iii) emerging technologies and potential commercial applications of bio-inspired IQA, and (iv) how these advancements will shape the future of visual technology, from photography and video to medical imaging and beyond. Participants will gain a comprehensive understanding of how bio-inspired computation is revolutionizing image quality assessment and optimization, ushering in a new era of visual technology.
Biography: Dr. Sos Agaian is a Distinguished Professor of Computer Science at the Graduate Center and the College of Staten Island, CUNY. Before joining CUNY, he was the Peter T. Flawn Professor at the University of Texas at San Antonio. He also served as a Visiting Professor at Tufts University and a Lead Scientist at Aware, Inc. in Massachusetts. His research spans computational vision, machine learning, AI, multimedia security, remote sensing, and biomedical imaging. Dr. Agaian has received funding from NSF, DARPA, Google, and other agencies. He has published over 850 articles, 10 books, and 19 book chapters and holds 56 patents/disclosures, many of which have been licensed. He has mentored 45 PhD students and received multiple awards for research and teaching, including the MAEStro Educator of the Year, the Distinguished Research Award, the Innovator of the Year, the Tech Flash Titans-Top Researcher Award, and recognition as an Influential Member of the School of Engineering at Tufts University. He is an Associate Editor for several journals, including the Image Processing Transaction (IEEE) and IEEE Transaction of Cybernetics. He is a fellow of the Society for Imaging Science and Technology (IS&T), the Optical Society of America (SPIE), the American Association for the Advancement of Science (AAAS), Institute of Electrical and Electronics Engineers (IEEE), The Asia-Pacific Artificial Intelligence Association (AAIA), and a Foreign Member of the Armenian National Academy. He has delivered over 30 keynote speeches, 100 invited talks, and co-founded/chaired over 200 international conferences. He has also been a Distinguished IEEE Systems, Man, and Cybernetics Society Lecturer.
Prof. Michael Wang
Great Bay University, China
(Keynote Speaker)
Speech Title: Embodied Robot Skills and Good Old Fashioned Engineering
Abstract: With the advent of the large language models (LLMs), “end-to-end” large robot action models begin to blossom in very recent years with enormous enthusiasm for making humanoids and other robots. Initial results of recent advances seem promising, and major collaborative efforts are underway to collect demonstration data. But where do these large robot action models lead us to?
I will focus on manipulation skills that are necessary for robots to perform action tasks with needed intelligence in a home or a factory, especially for car assembly or electronics assembly. I'll review and share my perspectives on current trends in robot action task definition, data collection, and experimental evaluation. I argue that to reach expected performance levels in “robot skill acquisition”, we’ll need “good old fashioned engineering”. In industrial automation, an action process is broken down by engineers to a degree that they figure out how it works—the parameters of process—and write down the recipe; then robots are programmed to follow it. This is very similar to the approach of rule-based or symbolic AI (now known as GOFAI, “good old-fashioned AI”). But coming up with hard-coded parameters that capture the processes of general nontrivial physical tasks proved too hard, just as for GOFAI in problem-solving for actual, nontrivial problems. In contrast, humans can be routinely trained to “acquire” dexterous manipulation skills, often using specific tools. Underlining the skill set is delicate hand and eye coordination enabled by multi-modality sensing combining vision and hand tactile perception.
For robot skill acquisition, I argue that we need systematic approaches to combine GOFE and learning, in a modular rather than monolithic framework, such as MANIP, that integrates engineering and modularity with learning. Within this framework, I will examine features of human skin tactile properties with special emphasis on the characteristics which are vital in the design of robot hands. While the role of various mechanoreceptors in the human hand are well understood in relation to the stimuli like force, position, softness, and surface texture, the necessary engineering features of a robot tactile sensor, such as, spatial and temporal resolutions, force sensitivity and dynamics granularity, are yet to be addressed or explored, if we take human hand as a suitable tactile model for achieving human-like levels of robot manipulation skills.
Biography: Michael Yu Wang is a Chair Professor and the Founding Dean of the School of Engineering of the Great Bay University. He has served on the engineering faculty at University of Maryland, Chinese University of Hong Kong, National University of Singapore, Hong Kong University of Science and Technology, and Monash University. He has numerous professional honors–Kayamori Best Paper Award of 2001 IEEE International Conference on Robotics and Automation, the Compliant Mechanisms Award-Theory of ASME 31st Mechanisms and Robotics Conference in 2007, Research Excellence Award (2008) of CUHK, and ASME Design Automation Award (2013). He was the Editor-in-Chief of IEEE Trans. on Automation Science and Engineering, and served as an Associate Editor of IEEE Trans. on Robotics and Automation and ASME Journal of Manufacturing Science and Engineering. He is a Fellow of ASME, HKIE and IEEE. He received his Ph.D. degree from Carnegie Mellon University.
Prof. Jixin Ma
University of Greenwich, UK
(Keynote Speaker)
Speech Title: Temporal Aspects of Artificial Intelligence
Abstract: The notion of time plays an essential role in modelling natural phenomena and human activities concerning the dynamic aspect of the real world. Virtually, most knowledge in the universe of discourse is time-dependent and suitable methodologies have to be developed to cope with this. In particular, temporal reference is an idea deeply integrated in human common-sense, and many Artificial Intelligence systems need to deal with the temporal dimension of information, the change of information over time and the knowledge about how it changes.
One of the simplest, and the most important, human temporal enterprise is to handle time dependent information involving questions such as "when the car was collected from the garage", "what happened after the shop had been closed", and "until when was the suspect away from home" and so on. In fact, time seems to play the role of a common universal reference - everything appears to be related by its temporal reference, although the temporal reference may be absolute, e.g., "The shop opens at 9:00 am", or just relative, e.g., "He went back to his office after sitting in the garden for about 15 minutes.
Various areas in the domain of Artificial Intelligence require temporal representation and reasoning, including Prediction/Planning, Diagnosis/Explanation, Pattern Recognition, Industrial Process Control, Temporal Database Management, Historical Reconstruction, Natural Language Understanding, etc.
Biography: Dr Jixin Ma is a Full Professor of Computer Science (Artificial Intelligence) and the Director of PhD/Postgraduate Research Programme in the School of Computing and Mathematical Sciences at University of Greenwich, U.K. He has been the Director of the Centre for Computer and Computational Science and the Lead of Artificial Intelligence Research Group. Professor Ma is also a Visiting Professor of Beijing Normal University, Hainan University, Anhui University, Zhengzhou Light Industrial University and Macau City University. Professor Ma obtained his BSc and MSc of Mathematics in 1982 and 1988, respectively, and PhD of Computer Sciences in 1994. His main research areas include Artificial Intelligence, Data Science, and Information Systems, with special interests in Temporal Logic, Information Security, Machine Learning, Case-Based Reasoning and Pattern Recognition. Professor Ma has been a member British Computer Society, American Association of Artificial Intelligence, ACIS/IEEE, World Scientific and Engineering Society, and Special Group of Artificial Intelligence of BCS. He has also been the Editor of several international journals and international conference proceedings, Conference/Program Chair, and Invited Keynote Speakers of many international conferences. Professor Ma has published more than 200 research papers in peer-reviewed international journals and conferences.
Prof. Seokwon Yeom
Daegu University, South Korea
(Invited Speaker)
Speech Title: Drone-based thermal object tracking for search and rescue missions
Abstract: Infrared thermal imaging is useful for human body recognition for search and rescue (SAR) missions. This speech addresses thermal object tracking for SAR missions with a drone. The entire process consists of object detection and multiple-target tracking. The YOLO detection model is utilized to detect people in thermal videos. Position measurements in two consecutive frames initialize the track. Tracks are maintained using a Kalman filter. A bounding box gating rule is proposed for the measurement-to-track association. The track-to-track association selects the fittest track for a track and fuses them. In the experiments, three videos of three hikers simulating being lost in the mountains were captured using a thermal imaging camera on a drone. Robust tracking results were obtained in terms of average total track life and average track purity.
Biography: Seokwon Yeom has been a faculty member of Daegu University since 2007. He has a Ph.D. in Electrical and Computer Engineering from the University of Connecticut in 2006. He has been a guest editor of Applied Sciences and Drones in MDPI since 2019. He has served as a board member of the Korean Institute of Intelligent Systems since 2016, and a member of the board of directors of the Korean Institute of Convergence Signal Processing since 2014. He has been program chair of several international conferences. He was a vice director of the AI homecare center and a head of the department of IT convergence engineering at Daegu University in 2020-2023, a visiting scholar at the University of Maryland in 2014, and a director of the Gyeongbuk techno-park specialization center in 2013. His research interests include Intelligent image and optical information processing, deep and machine learning, and target tracking.
Prof. Weinan Gao
Northeastern University, China
(Invited Speaker)
Speech Title: Integrating Learning-based Adaptive Optimal Control for Advanced Applications in Connected and Autonomous Vehicles
Abstract: The integration of learning-based adaptive optimal control techniques into connected and autonomous vehicle systems represents a significant advancement in the area of intelligent transportation. In this talk, we explore the methodologies and applications of learning-based adaptive optimal control that are transforming the operation of connected and autonomous vehicles within dynamic and uncertain environments. We provide an overview of the foundational principles of adaptive optimal control and reinforcement learning, explaining how these two fields can be combined to generate robust and efficient control strategies.
Biography: Weinan Gao received the Ph.D. degree in Electrical Engineering from New York University, Brooklyn, NY, USA. He is a Professor with the State Key Laboratory of Synthetical Automation for Process Industries at Northeastern University, Shenyang, China. Previously, he was an Assistant Professor of Mechanical and Civil Engineering at Florida Institute of Technology, Melbourne, FL, USA, an Assistant Professor of Electrical and Computer Engineering at Georgia Southern University, Statesboro, GA, USA, and a Visiting Professor of Mitsubishi Electric Research Laboratory (MERL), Cambridge, MA, USA. His research interests include reinforcement learning, adaptive dynamic programming (ADP), optimal control, cooperative adaptive cruise control (CACC), intelligent transportation systems, sampled-data control systems, and output regulation theory. Prof. Gao is the recipient of the best paper award in IEEE Data Driven Control and Learning Systems (DDCLS) Conference in 2023, IEEE International Conference on Real-time Computing and Robotics (RCAR) in 2018 and the David Goodman Research Award at New York University in 2019. He is an Associate Editor of IEEE Transactions on Neural Networks and Learning Systems, IEEE/CAA Journal of Automatica Sinica, Control Engineering Practice, Neurocomputing and IEEE Transactions on Circuits and Systems II: Express Briefs, and a Technical Committee member in IEEE Control Systems Society on Nonlinear Systems and Control, IFAC TC 1.2 Adaptive and Learning Systems, and CAAI Industrial Artificial Intelligence.